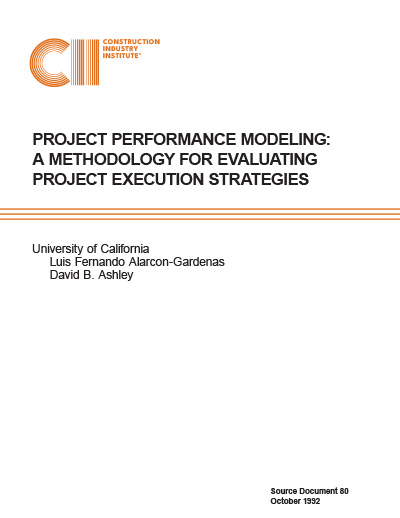
Project Performance Modeling: A Methodology for Evaluating Project Execution Strategies
The dynamic nature of today’s construction industry compels contractors and owners to seek strategies to improve performance. Intensive local and foreign competition for construction contracts, new alliances between owners and contractors, and in general, changing approaches to construction characterize ongoing industry changes. Under these conditions, it is difficult to predict implications of individual or combined construction execution strategies on project performance. Understanding these implications is key to successfully meeting the new challenges facing construction in the 90’s and on into the twenty-first century.
In response to these challenges, the Construction Industry Institute’s (CII) Project Team Risk/Reward (PTRR) Task Force developed a performance modeling methodology for application to individual projects. The model combines experience captured from experts with assessments from the project team. The methodology consist of a conceptual qualitative model structure, and a mathematical model structure. The conceptual model structure, called General Performance Model (GPM), is a simplified model of the variables and interaction that influence project performance. The mathematical model uses concepts of cross-impact analysis and probabilistic inference to capture the uncertainties and interactions among project variables.
Project options such as organizational structures, incentive plans, and team building alternatives have been incorporated into the model knowledge base. The GPM allows management to test different combinations of project execution options and predict expected cost, schedule, and other performance impacts. The methodology provides a systematic and structured process for a project team discussion on relevant planning issues in a project.
Study Objective
The objective of the study is:
To develop a new methodology for capturing and formalizing construction experts’ knowledge in such a way that a general model can be developed to analyze project performance. |
In order to address shortcomings in available methodologies, several desirable characteristics of the methodology were established:
- The evaluation of project performance should be addressed by utilizing multiple performance criteria and a flexible weighting procedure for evaluation.
- The methodology should evaluate different execution strategies, individually and combined.
- The modeling approach should include procedures to disaggregate the problem and capture expertise from construction experts at different levels.
- Uncertainties and cause-effect relationships among model variables should be an explicit part of the structure.
These characteristics are all incorporated into the proposed modeling methodology and are discussed in this Source Document.
Study Overview
The initial research effort was focused on exploration of potential research support areas. A literature review covered construction project performance with emphasis on modeling and evaluation, as well as other technical aspects that were expected to provide research support. Some of the topics covered in the literature review were: decision analysis, reasoning under uncertainty, representation schemes, modeling for multi-objective decision-making, group decision-making, knowledge acquisition, bias-reduction techniques, cognitive requirements for human problem solving, inference methods for influence diagrams, and hardware and software alternatives for computer analysis.
A pilot study performed early in the study tested concepts of a proposed modeling methodology that included most of the desired model characteristics enumerated above. The pilot study focused on the evaluation of incentive plans and resultant impacts on construction performance. This preliminary study demonstrated the methodology feasibility and helped define the final research tasks and methodology. It showed the need to systematize the knowledge acquisition process to prevent biases. It also suggested that an emphasis on cognitive aspects of modeling and problem solving was required to develop a methodology that effectively supports management decision-making.
A systematic knowledge acquisition process was followed in the research activities. A conceptual performance model was developed using as a starting point the model developed in the pilot study. Three options areas; organizational structure, incentive plans and team building alternatives; were selected and characterized.
The CII Project Team Risk/ Reward (PTRR) Task Force developed a performance modeling methodology that can evaluate the impact of project execution strategies on project performance to support project planning decisions. The project combines experience from experts with assessment of the project team and organizes it in a causal model where the team specifies the performance elements to be evaluated. Some features of the methodology:
- Predictive capabilities to help establish performance targets
- Incorporates risks and uncertainties, usually present in construction projects, in the analysis
- Allows a more comprehensive evaluation than currently available methods which are limited to one or two performance measures
- Allows the evaluation of the effects on project performance of two or more management options acting simultaneously
- Provides sensitivity analysis capabilities to identify the most important variables for project performance
- Provides not only results but exploratory capabilities through the model causal structure. It can help management obtain a better understanding of factors that affect performance, to identify opportunities for improvement, and then take effective actions.
The mathematical model developed in this research is characterized by an innovative use and integration of previous research from dissimilar fields such as Futures Research and Probabilistic Algorithms. A number of adaptations and extensions to previous research were carried out in this report. Some of the tasks accomplished in this area are:
- Adaptation of the Cross – Impact analysis events’ structure to represent the performance level of the GPM variables.
- Modifications of the original Cross – Impact algorithm to incorporate characteristics of the GPM events and improve computer processing performance.
- Design of the analysis approach to predict performance from the Cross – Impact Analysis results.
- Modification and adaptation of an algorithm to perform probabilistic inference to combine probabilistic information obtained from model results. The proposed algorithm allows the evaluation of the simultaneous effect of multiple options on project performance.
- Simplification in the knowledge acquisition demands of the traditional Cross – Impact methodology through the introduction of the concept of patterns.
The planned computer implementation of this methodology can have substantial benefits. The prototype version developed for this report has allowed the testing of the algorithms and has provided recommendations for final implementation. Benefits are:
- Reduction in analysis effort
- Inexpensive exploration of execution strategies
- A new door to innovation by providing a tool to systematically evaluate new and untried construction strategies
The methodology provides a framework for capturing and formalizing construction experts’ knowledge and integrating research information. A systematic methodology for knowledge acquisition in the modeling process supports one of the major difficulties found in practice for the development of this type of model. The knowledge is stored in modules that are self-contained and independent. Some of the features resulting from the above characteristics are: (SD-80, p. 208)
- It facilitates isolation and capture of expertise. Each expert can contribute his/her own expertise in a localized area.
- It automatically introduces causal relationships that describe the interactions among project variables.
- It can be easily updated without altering the model structure.