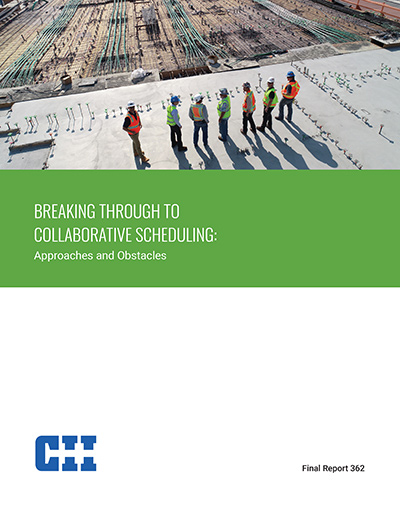
Breaking through to Collaborative Scheduling: Approaches and Obstacles
Traditional scheduling methods deliver mixed results. CII benchmarking data show that more than half of all projects are delivered behind schedule and the majority of megaprojects are delivered late. When measured in terms of duration and sequencing, the execution of construction activities is rarely a success if project participants simply follow a master schedule developed at the beginning of the project by using the Critical Path Method (CPM).
Capital projects across all industry sectors involve a number of interconnected processes and functions, including planning, financing, designing, procuring, constructing, and commissioning structures for business purposes. Given the uncertain and interdependent environment of construction projects, a single person (the scheduler) cannot perform reliable scheduling, even if he or she defines detailed work packages well in advance of execution.
To address these issues, the industry has observed that collaborative scheduling practices have emerged to address the current shortcomings in capital project management. Although CPM has been the capital project industry’s standard for scheduling, other industries are using new, apparently more collaborative methods. Given the power of collaborative scheduling (CS) to address the industry’s pressing needs, the construction industry needs guidance to encourage project participants to implement it.
CII asked Research Team 362 (RT-362), Challenges and Opportunities to Promote Collaborative Scheduling, to answer the following essential question:
What are the drivers of and obstacles to the development and implementation of collaborative scheduling?
As a starting point, the research team adopted the following definition of collaborative scheduling:
Collaborative Scheduling is a comprehensive process that aligns and engages stakeholders throughout the life cycle of the project in order to coordinate activities and resources on a project and achieve its goal.
RT-362 examined collaboration in project scheduling and identified opportunities for improvement and barriers to CS, based on an industry analysis. The team gathered its findings into a series of strategies that could be applied to implement CS across an organization. These strategies are the focus of Chapter 2, a high-level introduction to CS for organizations that are willing to adopt collaboration.
The team also developed a Maturity Model for Collaborative Scheduling (MMCS) to organize the team’s recommendations for implementing CS. Chapter 3 explains how the maturity model identifies the current state of a project’s CS. The MMCS provides practical recommendations and examples of how projects can be improved by increasing collaboration across five pillars: scheduling significance, planners and schedulers, scheduling representation, goal alignment with owner, and communication. Chapter 3 also offers scenarios that illustrate how CS can be applied to current construction projects, and Appendix A contains the MMCS questionnaire and scoring rubric.
Finally, as Chapter 4 describes, RT-362 developed ways to understand the benefits of and barriers to CS implementation across multiple projects. It identified collaboration improvement opportunities at the project level in terms of five key performance indicators (KPIs): cost, schedule, safety, quality, and teamwork.
RT-362 categorized 23 collaborative scheduling practices into five pillars, which are the foundation for its maturity model. The team identified these model components through focus group discussions with SMEs and by developing affinity diagrams to organize the clusters into pillars and lanes (FR-362, p.10).
The team established scoring criteria cut-offs for each level of implementation maturity, based on the results of projects provided by survey respondents. The researchers analyzed each project by using the k-means clustering algorithm. This analysis used a total of 241 valid responses (FR-362, p.10).
RT-362 evaluated the five pillars and identified two as the most important to enable organizations to achieve gold status on CS: Goal Alignment with Owner and Scheduling Significance. The team then evaluated the attributes within each pillar and ranked them by using Multi-Objective Decision Analysis (MODA, a utility-based analysis technique) and the Delphi Method. Within the Goal Alignment with Owner pillar, Expectations bears the most weight in the process of achieving Gold. In the Scheduling Significance pillar, Culture is most important. The figure shows the weights for each pillar and attribute that the team identified (FR-362, p.11).
A chain of CS practices can be used in sequence to improve project performance. The figure illustrates how practices can be aligned to improve the performance of the five KPIs defined in the project, an analysis that resulted from using Chow-Lee trees (FR-362, 98).
The figure illustrates the most effective lanes for each pillar, relative to that pillar’s influence on the KPIs. For instance, the team identified having a Planning Mindset in the project as the most important lane in the Planners and Schedulers pillar because it is one of the top five lanes overall and affects both the Safety and Teamwork KPIs (FR-362, in press).
The three most effective practices were grouped under two pillars: Goal Alignment with Owner (green in the figure) and Scheduling Significance (purple). The Expectations and Interaction lanes under Goal Alignment with Owner were in the top three positions for the KPIs, while Scheduling Significance’s Visibility lane affected every KPI but Safety within the top three levels, and its Culture lane affected the Teamwork KPI at the top level (FR-362, in press).
As the figure shows, the 241 survey respondents perceived nine CS practices as consistently implemented, 21 as not consistently implemented, and two as consistently not implemented. They also rated each practice as having a high or low impact (FR-362, p. 51).
Final Report 362 explains how the team identified which CS drivers were most frequently mentioned in the interviews by applying qualitative analyses that used coding schemes. The report discusses these drivers in greater detail, showing how they support the model recommendations and augment the findings obtained from the team’s quantitative analysis of the survey results (FR-362, in press).